Extracting Frequent Patterns From a Volunteered Tick Bites Collection
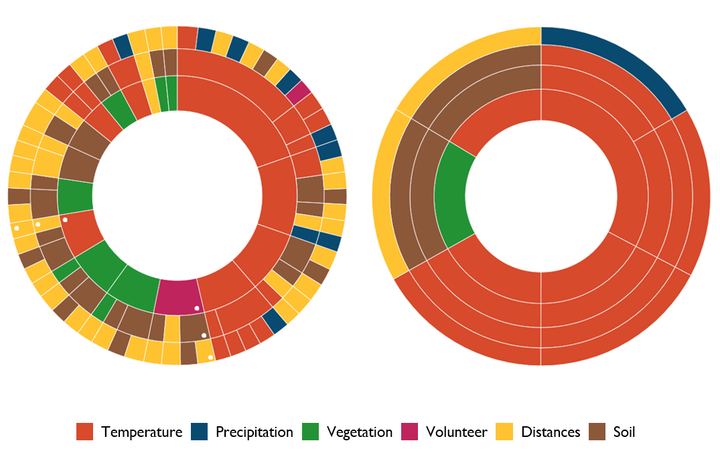
The first paper was published in 2016 in a special issue of Transactions in GIS on the Role of Volunteered Geographic Information in Advancing Science. A year before, a very enthusiastic and naïve self had received a collection of 35,000 volunteered tick bites provided by the RIVM. I had some experience working with volunteered data because in my previous research group, I worked in several projects using this type of citizen-contributed information. Hence, classical problems attributed to citizen science data, such as spatial representativity, spatial inaccuracies, or noisy observations, were not strange to me. However this time it was different, since the goal was to consider these problems, plus later on analyzing the dataset assessing whether we could pick a signal from it using machine learning algorithms.
Back then, we were not really sure on what was representing the volunteered tick bites collection. This prompted a number of research questions that fueled discussions among several specialists in the fields of zoonotic diseases, ecology, and epidemiology. Questions such as what is the tick bite collection actually monitoring?, are the volunteered reports containing a substantial amount of spatial inaccuracy?, or how to validate the volunteered data collections?, were important, since the remaining of the thesis would build at the top of these findings. After some debate, we used a framework from risk assessment, in which the tick bite risk (R) is a product of the tick hazard (H) and the human exposure (E) to ticks in a location. Or, in short, R = H x E.
We carried out an exploratory data analysis to assess which is the component above represented by the tick bites collection. We devised an array of 39 environmental, weather, and human features that we thought could help at characterizing the problem, and we modelled these data using a well-known frequent pattern mining method: Apriori. This algorithm explores our enriched tick bite reports with its 39 features to find combinations of variables that are frequent in our data. The image above shows a visual representation of the found patterns. The ring maps reveal that the patterns are strongly influenced by distance and temperature features. However, a closer inspection (in the article) of the temperature features shows that the tick bites tend to occur when temperatures crosses a threshold (e.g. today is higher than 20°C) rather an accumulation of temperature over a period, as it happens with the modelling of other phenological events.
These results suggested that the tick bites phenomenon could be more strongly influenced by human exposure (E) than to the existing tick hazard (H). Thus, after finding traces of both components, we concluded that the volunteered tick bites collection was representing the tick bite risk (R).
The process described above ensures that there are certain recurrent patterns in our data, but were these conditions real or just a product of the citizens' sampling?
Validation is challenging when working with volunteered data, because usually there is no reference dataset to compare with. In this case, a dataset was needed to verify whether the tick bites collection contained information intrinsic to ticks or tick bites, or it was just a product of randomness. We generated artificial locations of tick bites (taking care that they are not too close to the original ones), and we enriched them with the 39 features. After this step, we applied Apriori to extract frequent patterns in this dataset. The comparison of the artificially generated patterns and the original patterns revealed no coincidence between them, which means these are not product of random sampling.
The main conclusions of this study are that the volunteered reports…:
- …shared by thousands of citizens do contain information with scientific value.
- …are representing the R component, thus opening the door to creating tick bite risk maps.
- …seem to deem as more relevant human factors rather than the seasonal accumulation of weather variables.